A deep dive into the world of gene variants, their significance, and the potential to transform our understanding of epilepsy.
Setting the Stage: Understanding Gene Variants in Epilepsy
Epilepsy, a neurological disorder marked by sudden recurrent episodes of sensory disturbance, has long puzzled the medical community. With the advent of modern genetics, we’re inching closer to understanding its genetic origins. While we previously ranked epilepsy genes based primarily on direct observations, sequencing, and published literature, a revolutionary method promises to deepen our understanding of the genetic causes of epilepsy.
A New Approach: Leveraging the AlphaMissense Database
Most of our current understanding of the effects of gene variants on the appearance of epilepsy in patients relies on genetic testing. If a patient with epilepsy has a variant in a gene known to be associated with epilepsy, we classify it as likely pathogenic. This means that our understanding of epilepsy can only move as fast as we are able to diagnose cases of epilepsy and sequence those genomes. However, the innovative minds at Google have adapted their AlphaFold algorithm to create AlphaMissense, a database of all human proteins and their possible genetic mutations. AlphaMissense is “cataloging the effects of 71 million missense mutations” to help researchers investigate their impacts on human health. AlphaFold itself is an AI used to predict a protein’s 3D structure from its amino acid sequence and is independent of traditional literature. Recent publications, such as “Accurate proteome-wide missense variant effect prediction with AlphaMissense” (PMID: 37733863), herald its potential. But with every advancement comes new questions.
Cracking the Code: The Missing Pathogenicity Assessments
How many of the variants that have been identified are actually pathogenic? Of the 71 million missense variants identified by AlphaMissense, 32% are predicted to be possibly pathogenic. On the other hand, ClinVar, a database that stores the measured assessment data on gene variants, currently marks only 8% of its observed variants as pathogenic. Between the AlphaMIssense predicted pathogenic variants and the directly measured variants in ClinVar, there is a whopping 24% difference in the expected fraction of pathogenic variants.
Why is there such a huge difference and where are these missing pathogenic variants?

The Enigma of VUS: A Closer Look at Variants of Uncertain Significance
The answer might lie in what are called Variants of Uncertain Significance (VUS), a category of variants in ClinVar that has intrigued geneticists for years. VUSs are gene variants observed in patient genomes that clinicians have not been able to assign as either pathogenic or benign. Their exact role in pathogenicity remains ambiguous and more in vivo testing is required to make a final decision, but this type of assessment takes significant time and investment to complete.
How can we estimate the extent of pathogenicity in the group of VUSs without this in vivo data?
There are two differing calculations that we might make: (1) variants are evenly distributed across all genes in the genome or (2) they are concentrated in genes critical to disease onset. If variants are spread evenly across all genes in the genome, and based on the difference between AlphaMissense and ClinVar pathogenic assessment, we could speculate that about 24% of VUSs are pathogenic. However, we know that non-essential genes have a lower chance of representation in the AlphaMissense data because this database utilizes evolutionarily-conserved sequences as a major component of its classifier. Therefore, we would expect that VUSs would have a lower probability of showing up in non-essential genes, so the share of these 24% pathogenic variants may be significantly higher in essential genes that cause disease. A conservative approach suggests that maybe half of the VUS in ClinVar might be pathogenic.
AlfaFold's Contribution: Strengthening the VUS Assertion
To further delve into this hypothesis, we turned to AlphaFold. Using its detailed gene structures, our team embarked on a biophysical exploration. By analyzing a subset of genes associated with epilepsy, we sought to determine if protein stability could hint at pathogenicity.
Based upon recent observation that most missense variants miss-localize due to protein instability (PMID: 37732209), we undertook a Gibbs Free Energy analysis of a subset of genes involved in epilepsy to determine if protein stability could provide prediction of pathogenicity. We chose a 5 member subset of the 515 epilepsy genes that were sourced from ClinVar and publications listing the terms “epilepsy”, “epileptic” or “seizure” as associated with a gene activity. These were STXBP1, SCN1A, SCN2A, GAA, and SYNGAP1. Each of these genes had at least 10 variants listed as pathogenic in Clinvar, and some of these genes had many more (e.g. SCN1A at 719 pathogenic variants with 135 supported by multiple submitters). Selecting the most-supported variants (e.g. SCN1A has 20 pathogenic variants reported as pathogenic at least 6x in ClinVar, and 20 benign variants seen at least 37x in GnomAD), a diagnostic curve was generated and when applied to VUS, the levels of possible pathogenicity were found to range from 29% to 56%. Comparison of VUS calls in AlphaMissense and Gibbs Free Energy gave the same Possibly Pathogenic call 76% of the time.

List of Genes for ClinVar-Supported Diagnostic Curve Generation:
GRIA2 | CASR | GABRG2 | TPP1 | CLPB | GRIN2B |
MFSD8 | POLG | ARX | MTOR | KCNMA1 | CACNA1A |
ADGRV1 | SLC2A1 | SCN9A | TUBB2B | PRRT2 | CLN8 |
ADGRG1 | CREBBP | MAPT | TCF4 | NHLRC1 | MEF2C |
GRIA3 | STXBP1 | GAMT | UBE3A | UBA5 | SYNGAP1 |
AARS1 | MECP2 | SLC6A8 | CACNA1C | WWOX | GABRA1 |
SHANK3 | PCDH19 | CLN6 | ALDH7A1 | KCNC1 | SLC13A5 |
EPM2A | GRIN2A | CLN3 | DNM1 | PHGDH | AKT3 |
GRIN2D | SMARCA2 | WDR45 | ASAH1 | GRIA4 | FOXG1 |
PNPO | DCX | PRKN | PAFAH1B1 | KCTD7 | GRIN1 |
Our findings from these investigations not only enhance our understanding but also challenge established guidelines.
From Data to Action: The Role of RapidGen Models
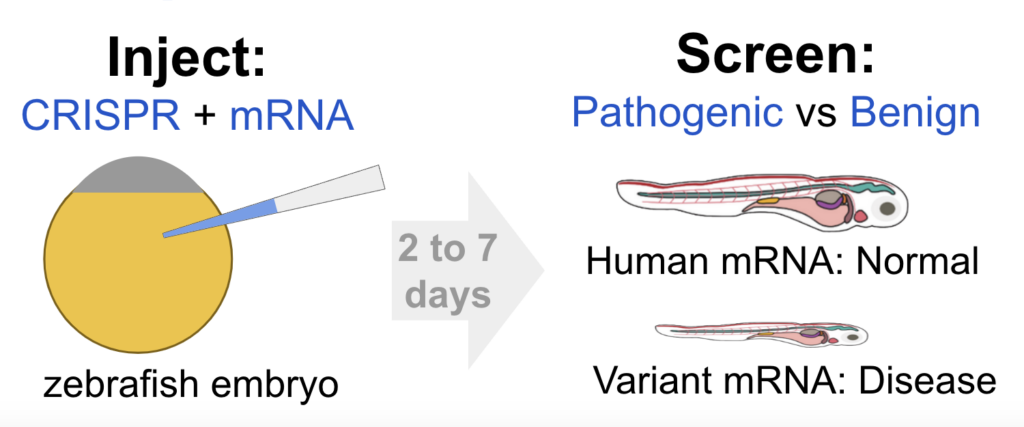
While data and insights are invaluable, real-world applications truly drive progress. To classify a Variant of Uncertain Significance as Likely Pathogenic, we need more than just bioinformatics. That’s where functional tests come into play, with methods ranging from test-tube binding assays to more complex animal models. At InVivo Biosystems, we are working to make animal modeling faster and more accurate. Our RapidGen Models provide early data on the pathogenicity of a gene variant target and are the first step toward a stable germline-edit model for detailed analysis and drug discovery. A major advantage of RapidGen Rescue Models is speed with initial insights into a disease in as quick as 2 to 7 days after injection.
Conclusion: The Road Ahead in Epilepsy Research
As we continue to decipher the genetic intricacies of epilepsy, tools like the AlphaMissense database and techniques like RapidGen screens pave the way for groundbreaking discoveries. With every gene variant we unravel, we move a step closer to a world where epilepsy is fully understood and, perhaps one day, entirely preventable.